We talk a lot about “good therapy” and “bad therapy” in the stuttering world. Speech therapy for people who stutter has a long, storied history of doing more harm than good, even when the therapist has the best of intentions. From the infamous Monster Study to “speech correctionism” to rigid approaches to fluency shaping to present day cultural and social judgments of disfluency, speech therapy is bursting at the seams with heavy baggage. Researchers and community advocates work in dedicated tandem to correct misconceptions and promote evidence-based perspectives, but it is difficult to reverse a deep current’s flow. Today, even popular SLP influencers endorse methods that are known to perpetuate ableist, shame-inducing practices. Hey, if these methods are so widely-used, it must be because they are so effective, right?
Amidst this ongoing challenge, we now we have a new tool to assist us with the hand-wringing question of what should I do with this stuttering client? Behold, artificial intelligence for the masses!
As a graduate course instructor gearing up to teach my first course in the ChatGPT era, I’ve wondered how students, novice clinicians, and clinical generalists might rely on generative AI to fill in knowledge gaps and generate ideas for therapy. Speech-language pathology has an enormous scope of practice with constantly updating research, making it practically impossible for an average clinician to keep up with best practices in any one area. And if you do try to dive in to refresh your knowledge, it’s difficult to find your footing in a sea of information.
If we start relying on tools like ChatGPT to filter through the noise and bubble up the key ideas, will this facilitate more evidence-based practice? Or will we be at risk of continuing the old mistakes, if that’s the bulk of the Internet’s corpus?
With just a few weeks until class starts, I decided to test this out myself!
This post covers:
- The importance of prompts quality and asking the right questions
- Treatment planning prompts examples and responses
- Evaluation of ChatGPT output
- How to ensure evidence-based practice while using AI
- Implications for clinical educators
Answers are only as good as your questions
Whether you’re starting with a human or an AI for your information source, the quality of the answer you receive is heavily dependent on the quality of question you provide.
Here’s the response from ChatGPT for the prompt, “What are some activities i can do with a client in stuttering therapy?”
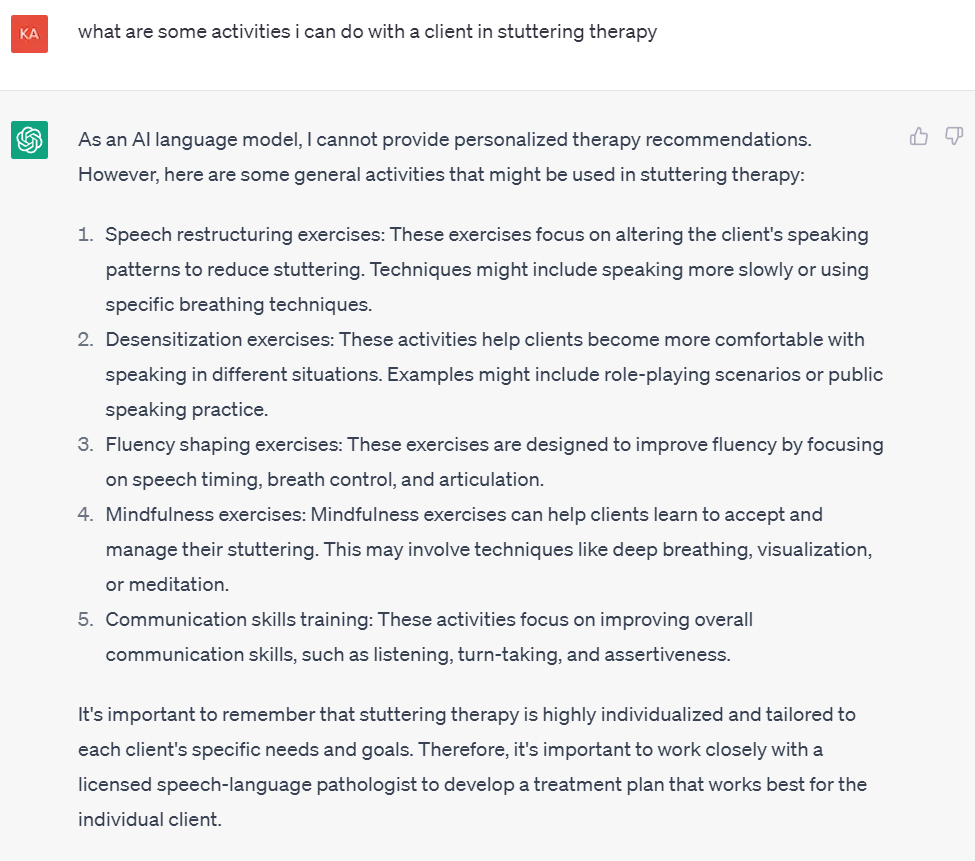
First off, props to the AI for acknowledging it is not a clinician. Disclaimers, check.
Overall, I would say this is a pretty fair representation of the breadth of activities that can be used in stuttering therapy. Fluency shaping is one of the oldest approaches to stuttering therapy, so I was not surprised to see that present. As a concept, desensitization became popularized after fluency shaping, but that term has been in the literature for at least a few decades, and I was glad to see it highlighted by the AI. I was pleasantly surprised that mindfulness was recommended in this curated list, because it is only relatively recently that mindfulness as a foundation for stuttering treatment has become more prevalent and established.
A major issue is that there is no mention of counseling, which is nowadays considered a critical component of evidence-based practice for stuttering therapy.
Considering the broad possibilities that exist within the scope of “stuttering therapy activities”, I was pretty impressed by the fact that this list is both comprehensive and concise. I was a bit surprised that reference to stuttering modification was not included somewhere in the response, given that term dates back to the early part of the 20th century and is still popular today. Those techniques would fall under “speech restructuring”, though, which is an accurate umbrella term.
While this answer could be a useful starting point for novice or generalist clinicians, most SLPs asking this question will likely need more detailed suggestions to put a real therapy plan together. Further prompts using any one of these initial suggestions should yield those results. However, this poses two issues inherent to the limitations of AI application for human experience.
First, the issue of what wasn’t suggested by the AI, counseling. If you use this tool as a first-step idea generator, and then narrow in on its suggestions, you could be at risk for having no counseling anywhere in your treatment plan. Of course, this is (one reason) why the AI advises you to use your human judgment, relying on your pre-existing knowledge to supplement or modify the AI’s suggestions. As long as you are trained and knowledgeable about stuttering therapy principles, you’re probably aware that counseling is important, and can use ChatGPT to get ideas on top of the items you already know are must-haves.
However, this leads to the second issue. The breadth of the initial suggested activity list is impressive, but these approaches are all quite different from one another. How does a clinician know which one(s) are most appropriate, and the implications of beginning with mindfulness first, or speech restructuring first, or something else? The AI cannot provide that context.
Evidence-based question-asking
To address the second issue, I decided to see if using a bit of EBP would help ChatGPT provide more nuanced suggestions. The 3Es is a conceptual framework designed to assist with therapy planning based on client needs and values. The model has some key terms that are designed to identify relevant therapy activities for specific therapy targets. What does ChatGPT provide if I ask it for ideas within different areas of the 3Es framework?
Below are the results, followed by evaluation of the responses.
EBP Prompt 1: Educate (3Es)
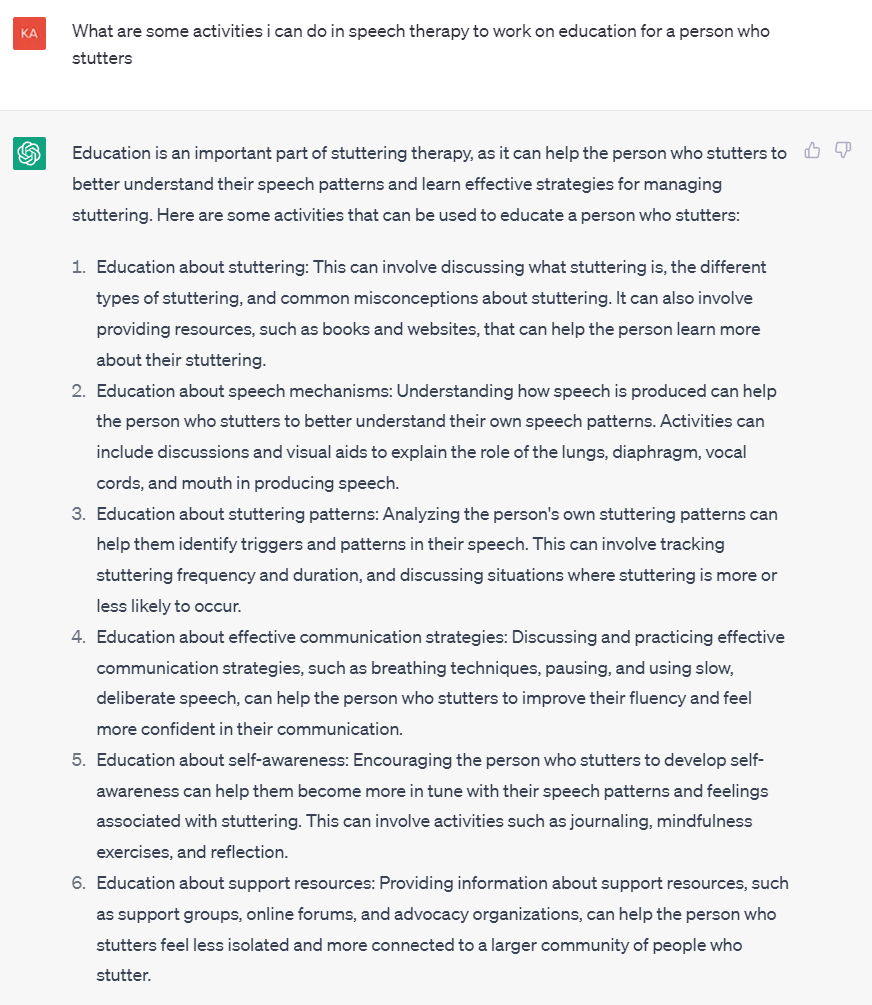
EBP Prompt 2: Empower (3Es)
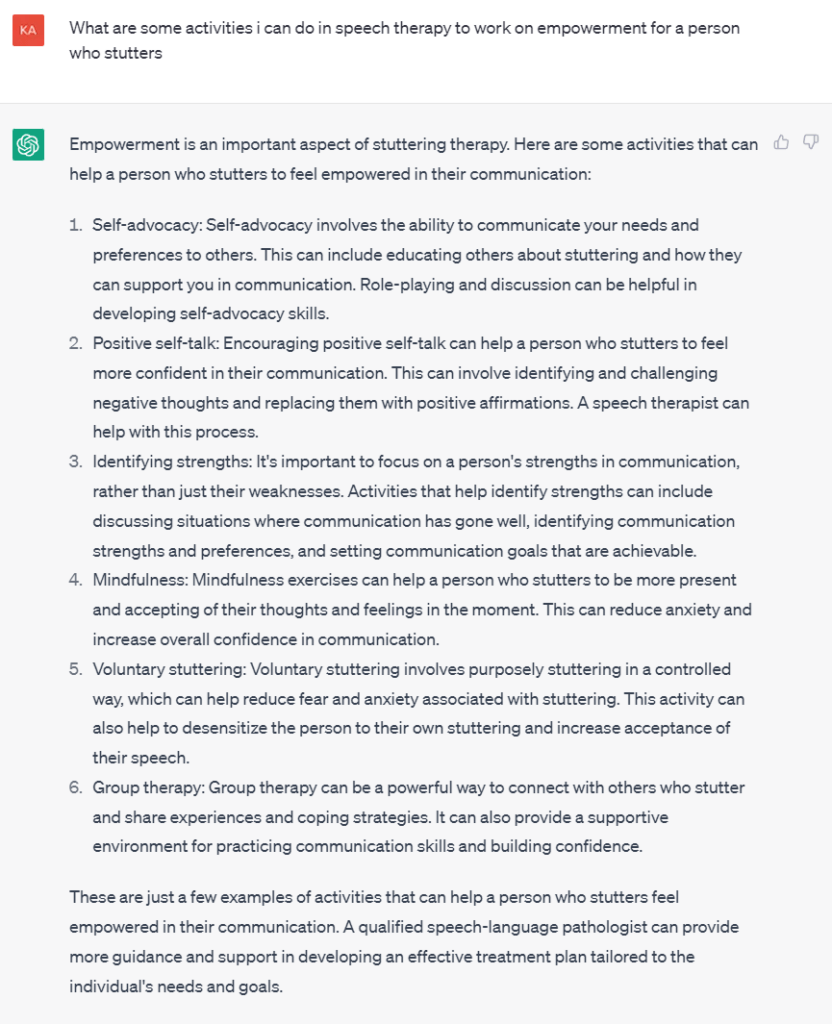
EBP Prompt 3: Ease (3Es)
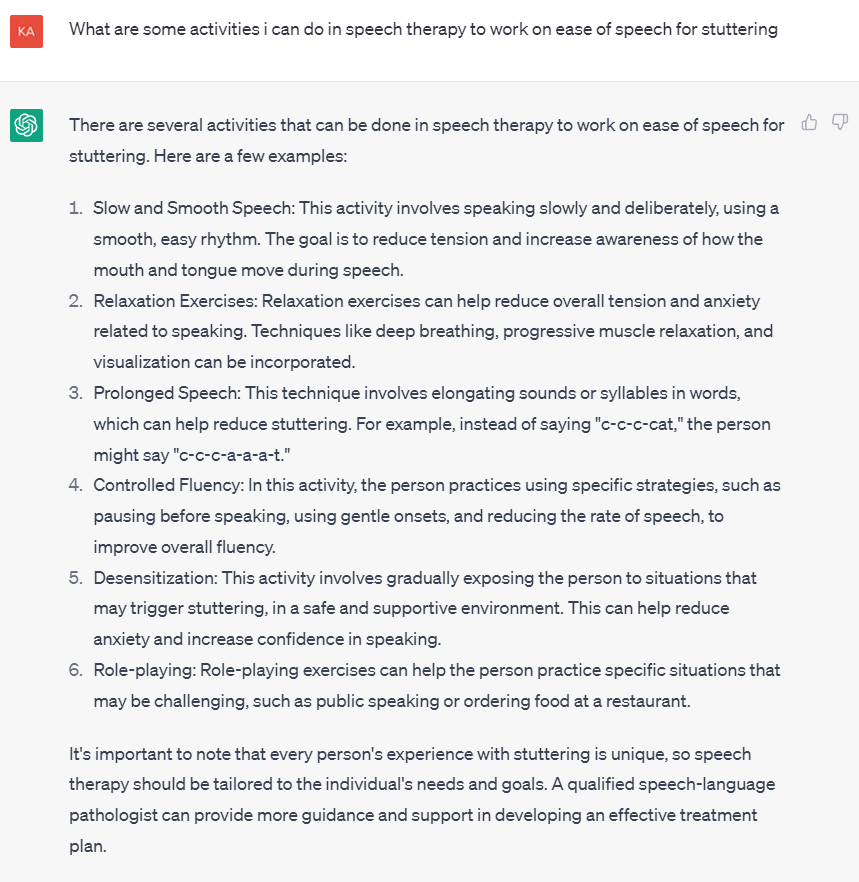
Evaluating ChatGPT
Honestly, I was pretty blown away by the responses for both Educate and Empower. All of those suggestions are solid, practical, and reflect current evidence-based practice and emerging research trends. Of course, these terms come from an evidence-based framework, so that may be a key factor for success: use current evidence themes to steer the AI where you want it to go.
To me, the suggestions appeared specific enough for a novice clinician to grasp the intent and purpose of the therapy activity, but broad enough to still require a final SLP flourish to become a therapy activity. By using key values- and theme-based terms in the ChatGPT prompt, the AI is able to generate an accessible list of possible therapy suggestions.
Conversely, I was disappointed at how one-dimensional the results were for Ease, in terms of the behavioral motor work suggestions. In this case, the EBP term did not suffice to send the AI in a direction that I felt good about clinically. I was glad to see the inclusion of role-playing and desensitization activities, although these are broader categories of behavioral intervention, versus a named technique or motor pattern. The actual recommended list of motor adjustments reads like a greatest-hits-of-things-that-don’t-work-so-well (or things that are often grossly misapplied, such as breathing and relaxation exercises). The AI-generated items reflect the most traditional, prescriptivist aspects of fluency shaping treatment. At best, this is just one limited slice of the possible approaches to physical speech modification, a problem of omission. At worst, and as is so commonly the case, these approaches can actually induce more shame, fear, and frustration around stuttering (and the uselessness of speech therapy), unless used carefully in conjunction with many other elements of therapy.
I was surprised and additionally dismayed that there wasn’t even a reference to alternative physical approaches like stuttering modification, which has had a strong presence in the literature for over half a century. The principles of the Normal Talking Model, used in Avoidance Reduction Therapy for Stuttering (ARTS) and popular among specialist practitioners, has no mention. (This did not surprise me, since the NTM parameters approach is probably the unintentionally best-kept secret of physical approaches to stuttering management.) It is understandable that the spontaneity/effort paradigm is nowhere to be found, given its extremely recent development, but the impact of this omission is equivalent to omitting counseling.
Conclusion: Generative AI is a historian-librarian, you are the scientist-clinician
I recently attended a webinar on the pitfalls and potential for AI, specifically in learning and developmental/educational settings. The excitement was palpable, but one commenter astutely observed that due to the fact that it is relying on the existing corpus of written knowledge, a tool like ChatGPT is currently only able to reflect past and most common practices. It is inherently not sensitive to trending innovations, or even recently established but less-commonly-practiced approaches.
Essentially, ChatGPT and similar AIs are very good at telling you what has been done, or what is often done, but it will not be able to indicate what is the most reflective of current evidence. This is a gravely serious issue in the field of stuttering therapy, which has such a history of perpetuating harm through practices that were intended to help.
ChatGPT’s responses are only as good as the questions that you feed to it. Thankfully, if your questions are evidence-based and specific, ChatGPT can be an incredible tool for planning treatment. Without this background knowledge or understanding of how pieces fit together, though, ChatGPT is just another list-generator. It will probably give you a mix of ideas that are great, and ideas that are terrible, or even approaches that are inherently contradictory (fluency shaping and avoidance reduction, for example). AI is a great tool for going treatment shopping, but the clinician must discern if the therapeutic whole will be lesser than, equal to, or greater than the sum of its AI-recommended parts.
So: would I use ChatGPT to plan treatment, personally? Absolutely I would. This type of accessible AI is not going away, and we need to learn how to work with it. ChatGPT has amazing potential to free up busy SLPs from the tedium of googling, leaving more time to do the critical thinking and empathetic aspects of treatment planning.
Final Notes for Instructors
ChatGPT has massive implications for clinical education, and we should keep this in mind as we are designing training for students and experienced professionals alike.
- We should spend relatively little time teaching students WHAT to do in therapy (the AI can do that). Instead, we should emphasize rationale, WHY we do certain things in therapy.
- We should also teach explicit rationale for why we DON’T do certain things in therapy, or why certain choices can be counterproductive if used improperly.
- We must teach about historical perspectives on different approaches to treatment, especially if modern evidence-based practices are a significant departure from what was popular in the past. Students and practitioners need to be able to immediately identify if the AI’s recommendations are reflective of old ways of thinking, or we risk doing real harm to clients, patients, and students in our care.
- We need to skill up on ChatGPT hard and fast ourselves, so that we can teach our students how to ask the right questions, and solidify their ability to identify AI responses that reflect the current best practice and best evidence.
The importance of teaching rationale has always been somewhat of a given in clinical instruction, but I cannot overstate how vitally important it is to carve out giant time in our curriculum for #2-4.
That’s about all the human-generated intelligence I can muster on this topic for now. I’m off to spend some more quality time with my robot student, to see what I can teach it, and what it can teach me!